Can AI Peer Review?
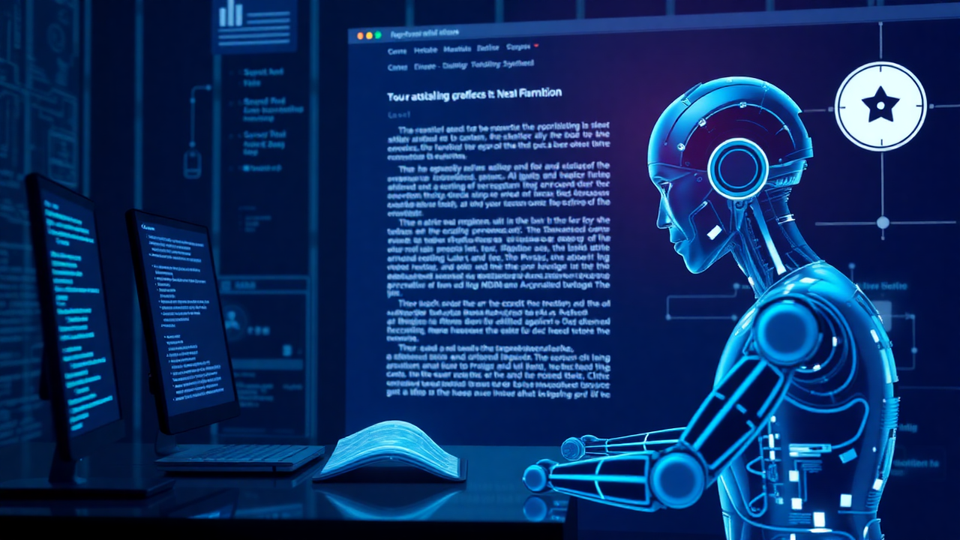
The short answer: no. (Long answer: Nooooooo.).
Can it assist with peer review? Possibly, but the process still needs extensive oversight by a human domain expert who is responsible for carrying out the main review tasks. The primary argument for using AI in peer review is one of efficiency, but such an approach (given the flaws inherent to generative AI) is really an argument for expediency (see Katz, 1992, for an explanation of why an ethic of expediency is one to avoid rather than embrace).
In our response here, we should note that we are specifically responding to attempts to use large language model (LLM) based generative "AI" systems (such as ChatGPT, Claude, Gemini, etc.) to perform peer review tasks on scholarly academic writing. LLM is what most people are referring to when they talk about AI in relation to writing. There are other AI systems that use machine learning that aren’t chatbots. To our knowledge, no one has yet developed any of these other AI approaches to consider peer-review—and most of the noise and hype about LLM-based systems like ChatGPT is drowning out news of other approaches in any case.
Writing for The Scholarly Kitchen, Christopher Leonard points to the massive increase in scholarly production as a key exigence for considering AI for peer-review; he notes that "every one of the estimated eight to nine million active researchers in the world" should be producing at least one peer-review report each year - but clearly most researchers are not doing so. Leonard notes that an AI can produce what appears to be a peer-review report in response to a given submission, but that "there is little or no comparison with previous literature, there is little or no evaluation of novelty, suggested references are prone to hallucinations, and there is a depressing tendency to rate everything as a minor revision." This list of limitations should be unsurprising, as they are essentially baked into the system—generative AI applications can only produce human-like language; they cannot provide any actual evaluation.
Turning to AI to deal with the shortage of peer-reviewers (as well as the lack of training of peer-reviewers) seems like a possible solution to the major challenges journal editors are facing. But in what sense can such a system be considered a peer? It does not have a knowledge base in the traditional sense: It's not a database—and it also does not know the difference between fact and fiction. It doesn't know anything at all. It certainly can make no claim to disciplinary expertise or understanding. It has no way of evaluating its own output, which is simply to produce plausibly human-sounding language.1 AI systems can provide some reasonable feedback and make suggestions for improvement, but employing them for these purposes should be a task undertaken by the authors, or possibly by editors who assist authors with developmental editing.
Leonard suggested that "The best use of LLMs in peer review today could be their use by the editor to cross-check human peer review reports and make sure nothing has been missed or downplayed before querying with the reviewer," which seems like a reasonable use but still requires a high degree of oversight by the editor. We worry that the temptation to use AI, even in this limited manner, will result in overworked editors delegating some or all of their authority to systems that are not at all designed to do this work. We think there may be a role for AI in the overall process of scholarly production, but it should be quite minimal in the peer-review phase, and its use should be paired with strong human oversight in every preceding step of the process. And of course, any AI use needs to be performed on a system that is local or that is institutionally owned in order to make sure the drafts of the submission are not shared with the AI production companies and other third parties that are capturing data sent to public AI chatbots.
One Possible Model for Using AI to Support Peer Review
In an essay posted on Nature Careers Community, Dritjon Gruda (2025) suggested that adding a generative AI tool can be helpful to one's review workflow, but their workflow model only adds it as a way to transform notes about the submission into a coherent and well-organized review that will be helpful for the author. Gruda's approach starts with a holistic scan of the abstract, introduction, methods, and results, making sure the submission is complete, ready for review, and does not present sufficient flaws to lead to an initial rejection. The second step is to make notes while reading; Gruda's process does this through voice recording on a voice-to-text app. The AI tool comes in when converting the notes and impressions into a good review letter.
This seems like a good model overall, although the reviewer still needs to carefully evaluate the AI-generated text to make sure it hasn't added anything that wasn't in the notes. One benefit of this approach is that the AI can be instructed to strike a collegial or helpful tone, rather than an overly critical one, even if the resulting recommendation is revise-and-resubmit. Gruda's model does assume that there is no value in producing a reader report if the submission is deemed a rejection in the first step—in my experience with writing studies journals, there is an assumption that a review should be informative and provide guidance to help the author improve, even if the submitted work is not ready for publication. If the goal is simply efficiency, then AI (with sufficient oversight) can potentially streamline the process; if the goal is to increase quality submissions in the future, then AI should probably have less input.
AI is Inherently Biased and Does Not Support Inclusive Editing Practices
Bauchner and Rivara (2024) argue that generative AI systems can be trained to perform peer-review tasks, which would be beneficial because "[a]rtificial intelligence could be developed that is less biased than peer-reviewers," apparently unaware that generative AI systems are inherently (and deeply) biased in fundamental ways—trying to reduce bias has been a serious challenge for the companies that produce their models, and it's fairly certain that such bias cannot be removed entirely. These authors also reference a study by Liang et al. (2023) that showed AI responses aligned with human responses around 30% of the time, which they described as "good agreement" because human peer reviewers tend to agree with each other about the same amount (according to the study's authors).
Our view is that the method of comparison (which used the same AI systems supposedly being evaluated to determine the overlaps) is somewhat flawed, and that the finding doesn't actually support the argument that AI systems would be capable of carrying out peer-review tasks. In a second part of that same study, 57% of authors claimed to find AI feedback helpful, but securing this kind of feedback could be done prior to submission. In contrast to the pro-AI stance, Chaturvedi (2024) enumerated the challenges with incorporating AI into peer-review processes, outlining issues with confidentiality, bias, accountability, and the potential for "compromising the value of human expertise."
Recommendation Against AI Use (in Peer Review)
When combined with the propensity of AI systems to invent and suggest non-existent works as worthy of citation, along with other irrelevant or incorrect responses, and the challenges of consent when it comes to providing submitters' texts to AI systems, we believe that peer reviewers should not use AI for any substantive part of the review process (and, honestly, it's much better to not use it at all).
References
Bauchner, Howard, and Frederick P. Rivara. 2024. Use of artificial intelligence and the future of peer review. Health Affairs Scholar, 2(5): https://doi.org/10.1093/haschl/qxae058
Chaturvedi, Aashi. 2024. AI in peer review: A recipe for disaster or success? American Society for Microbiology. https://asm.org/articles/2024/november/ai-peer-review-recipe-disaster-success
Chen, Yanda, Joe Benton, Ansh Radhakrishnan, Jonathan Uesato, Carson Denison, John Schulman, Arushi Somani, Peter Hase, Misha Wagner, Fabien Roger, Vlad Mikulik, Sam Bowman, Jan Leike, Jared Kaplan, and Ethan Perez. 2025, April 3. Reasoning models don’t always say what they think. Anthropic. https://assets.anthropic.com/m/71876fabef0f0ed4/original/reasoning_models_paper.pdf
Gruda, Dritjon. 2025. Three AI-powered steps to faster, smarter peer review. Nature Careers Community, 24 March. doi: https://doi.org/10.1038/d41586-025-00526-0
Katz, Steven B. 1992. The ethic of expediency: Classical rhetoric, technology, and the Holocaust. College English, 54(3): 255-275.
Lindsey, Jack, David Abrahams, Trenton Bricken, and Sam Zimmerman. 2025, March 27. On the biology of a large language model. Transformer Circuits Thread. https://transformer-circuits.pub/2025/attribution-graphs/biology.html
Leonard, Christopher. 2024, September. Is AI the answer to peer review problems, or the problem itself? The Scholarly Kitchen. https://scholarlykitchen.sspnet.org/2024/09/24/guest-post-is-ai-the-answer-to-peer-review-problems-or-the-problem-itself/
Liang, Weixin, Yuhui Zhang, Hancheng Cao, Binglu Wang, Daisy Yi Ding, Xinyu Yang, et al. (2023). Can large language models provide useful feedback on research papers? A large-scale empirical analysis. arXiv.org https://arxiv.org/pdf/2310.0178
Footnote
- Even so-called 'reasoning' models, which show their chain of thought (CoT) processes upon request, will provide plausible-sounding explanations for its reasoning, but those explanations are themselves generated text providing answers a human would expect and not actually a representation of its processes (Chen et al., 2025; see also Lindsey, Abrahams, Bricken, and Zimmerman, 2025, for a very detailed look at reasoning models—especially the section on 'Addition)'.